Difference between Data Analysis and Data Analytics: Which is Better?
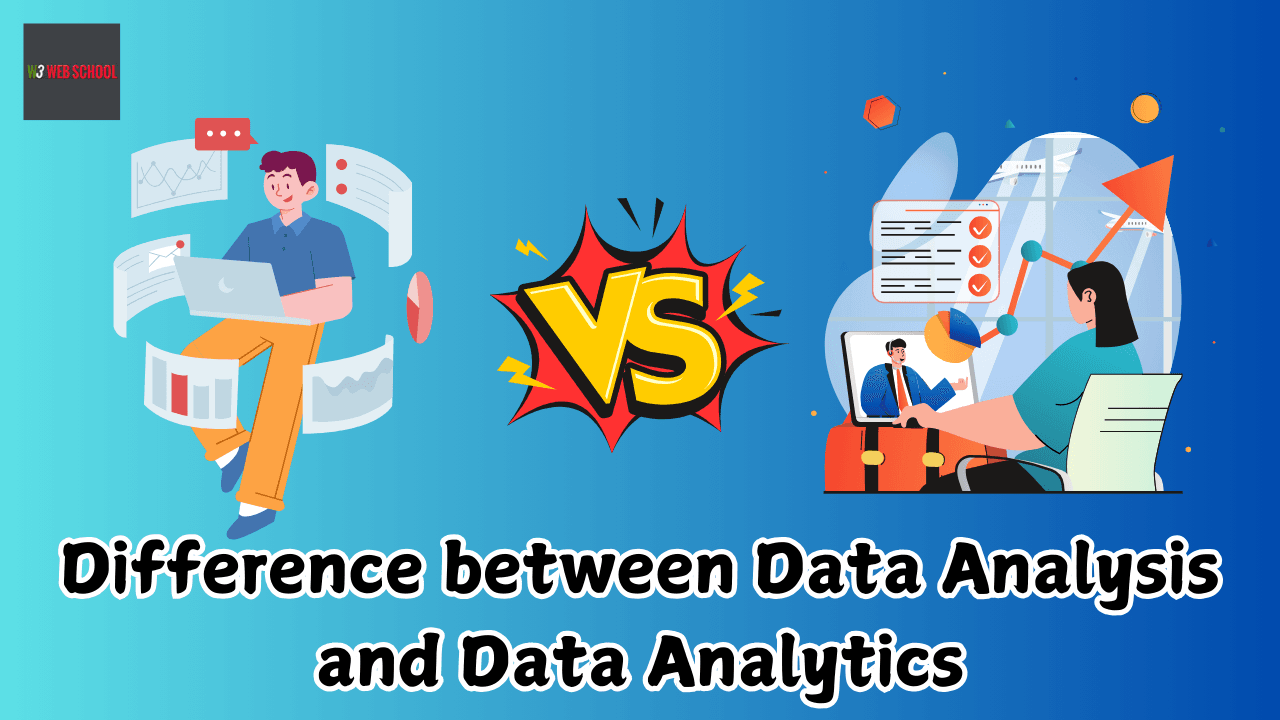
Are you still facing difficulty in stating the difference between data analysis and data analytics? The majority of businesses and individuals mistakenly believe that they are the same, although they are often used interchangeably. But they are not the same. They serve different purposes and make you confused while choosing the right one for your business growth.
The challenge arises when people fail to understand which one to pick for detecting and managing data-driven things. While data analytics focuses on advanced tools or techniques such as machine learning or predictive analytics, data analysis is highly focused on data interpretation to discover past data patterns and trends.
In this blog post, I will highlight the difference between Data Analysis and Data Analytics. Defining their concept and elaborate on their key characteristics, explore types and review which one is better.
Want to make data-driven decisions?
Enroll in W3 Web School’s Data Analytics Course to find the data patterns for making statistical predictions in 2025.
Table of Contents
Data Analysis vs Data Analytics: At a Glance
In today’s fast evolving data-driven landscape, most of the businesses highly depends on real-time data to make beneficial decisions. Although, bothe the terms often placed interchangeably and leave people confused which one to choose for better outcome according to their specific needs. Both of data analysis and data analytics serve different elements of the data process while they both involves working on huge volume of data sets.
If you have a clear understanding of both of them, you will be guided wisely in discovering which data field resonates with your specific needs and demands and alights highly with the aim of your business.
Reviewing raw data to discover information that can be employed is referred to as data analysis. Before evaluating the information to identify trends, patterns, or links, it should first be organized and cleaned. Depending on the real-time facts, the major purpose is to offer specific responses or address data errors. Reports and evaluations of previous events or incidents often use data analysis. It is basically about understanding what has happened in the past and what the data proof reveals.
Evaluating data employing more advanced technologies is part of a greater process known as data analytics. It makes predictions or recommendations with the use of advanced tools, techniques, statistical techniques, and algorithms. Through future pattern predicting along with assessing the past, data analytics helps businesses in making data-driven decisions. It requires more deeper comprehension, often focusing on revealing hidden data patterns or solving complex data issues. It uses advanced technology to boost business productivity and optimize methods.
Difference between Data Analysis and Data Analytics
Data analysis vs. data analytics, both these areas of Information and Technology are two different topics of the same discipline, generally correlative to each other. In the below section, I have tried to highlight every single element that differentiates data analysis from data analytics and aid you in clearing your understanding and doubts regarding them. This also helps you pick the right one based on your passion and career preferences for one specific professional field to begin your career.
Let’s get going.
The major purpose of data analysis is to resolve data errors and offer solutions to specific data queries by reviewing past history. It includes employing data interpretation to generate clear as well as practical insights. The key purpose of data analytics is to span over past data. Future trend prediction and data-driven decision-making are its major purposes. Data analytics aims to shape future decision-making by comprehending in-depth insights, whereas data analysis tries to gain insight into the past or present.
Data analysis has smaller scopes from conducting functions such as organizing, cleaning and interpreting significant data for gaining particular insights. This data field is highly focused on solving specific issues or responding to particular queries from the available data. On the other hand, data analytics has wider opportunities. From advanced technologies such as machine learning to predictive modeling, data analytics helps you discover in-depth insights and predictions about future trends and patterns. It frequently assesses more challenging and large-volume data sets for data-driven decision-making.
Data analysis is popular for following very simple processes. In order to make predictions, it generally involves in data collection, data cleaning and data evaluation at the end. With a focus on specific goals, the data structure is often linear.
On the other hand, data analytics is highly advanced and organized. Data cleaning, collection, analysis, modeling, and interpretation are among the steps that are connected. As data analytics requires advanced techniques such as predictive algorithms and machine learning, its structure is multi-layered and highly complex as compared to data analysis.
The functionalit of data analysis differs majorly from data analytics. The major function of data analysis is to discover hidden patterns and trends of past data. It deals with issues regarding “why something happed” and “what happened” depending on the past data. This data field is highly beneficial for making visualizations, report creation and generating insights.
On the other hand, data analytics offers more versatility and offers solutions to forthcoming data issues. Data analytics helps businesses in boosting the value of previous data, discovering new patterns for better initiatives in approaching 2025. From reducing business risks, making data-driven decisions to cost controlling, data analytics drive busines growth.
Data gathering, data cleaning, data interpretation, and instant reporting are the basic stages of the data analysis process. The sequence is simple and focused on findings from previous data. On the other hand, data analytics is highly complex process. Same processes or sequences are employed at first, but more advanced approaches such as algorithm application, and advanced data modeling are integrated later. In the process of data analytics, the sequence is specially designed to generate predictions and axctionable recommendations, often involving repeated analysis and steady advancement.
Data analysis helps you in cleaning, organizing, and visualising vital data employing basic statistical software and tools. For analyzing small volume of datasets, tols such as Excel, OpenRefine, Power BI applications, NodeXL, RapidMiner, KNIME, and SQL are used mostly.
More advanced technologies and tools are essential for data analytics inlcusing big data platforms, statistical tools such as Python, SAS, Google Analytics, ChartExpo, Tableau Public, R, Apache Spark, or Hadoop, and advanced machine learning techniques. In-depth data analysis, data prediction on huge amount of data sets, and automation are easily accessible by the use of these advanced data analytics tools.
There are three types of data analysis, they are Hypothesis analysis, Content analysis, and Regression analysis.
Data analytics are classified into four major categories and they are descriptive, diagnostic, prescriptive and predictive analytics. These different types of data analytics aid you in resolving complex business challenges while handling real-time data. Along with these four major types, there are subtypes also and they include statistical analytics and text analytics.
Common duties of a person from data analysis include data cleaning, report generation, statistical analysis, data preparation, visualization of trends, and reacting instantly to specific business demands. Presenting and interpreting data in a comprehensive way are the roles and responsibilities of a person concerning data analysis.
The role and responsibilities of a person concerning data analytics is more broader in data field. It helps in creating data models, integrating advanced statistical analysis, and making predictions depending on data. In this role, data analysts often focus on the advanced process of data optimization and providing businesses real-time recommendations.
Another major element of data analysis is known as descriptive analysis. The major objective is to explain previous data in order to figure out what and why it happened. It analyses previous data to detect data trends, patterns, and key indicators. This descriptive analysis process is employed by data professionals to develop real-time data reports and visualizations that help businesses predict past behavioral patterns. Data analysis does not support descriptive analysis. On the contrary, data analytics involves descriptive analysis as well as other forms of analysis that are more focused on the future and recommendations, such as predictive and prescriptive.
Both of the data analysis and data analytics hold different types of technical skills. The foundational skills essential for a person dealing data analysis are statistical skills and data management. The individual must be able to manage tools such as Exel, SAS, SQL and visualization tool such as tableau.
On the contrary, people dealing in data analytics field required to have skills in artificial intelligence, machine learning, programming languages such as R and Python and statistical modeling. Comprehending big data technologies, complex data structure and predictive analytical technologies are essential for data analysts dealing the analytical landscape.
Basic statistical techniques like mean, median, mode, and correlation are most frequently used in data analysis to assess hidden data. It prioritizes identifying and stating hidden as well as past data patterns. On the other hand, data analytics is comprised of techniques such as machine learning algorithms, regression analysis, and predictive modeling. Such advanced techniques aid businesses in detecting hidden data patterns, future trend predictions, and making real-time data-driven decisions. Data analytics needs specialized skills as compared to fundamental data analysis techniques.
Data analysis often performs quicker, focusing on assessing and reviewing the latest data and generating findings as fast as possible. Putting everything together or analyzing the latest or previous data is the major focus. On the contrary, data analytics operates over a longer time frame. It involves employing data predictive models, testing situations, and analyzing a huge volume of datasets, all of which take additional time periods. Deeper analysis and the creation of concepts or approaches that will impact future predictions are made simply by the prolonged time frame.
A statistical approach for making assumptions about a huge amount of population from a specific sample of data is known as inferential analysis. It helps businesses in understanding data patterns in the dataset or predicting future incidents. Inferential analysis is one of advanced methods used in data analysis.
Data analysis enables inferential analysis to come to a summary and make statistical predictions. On the other hand, data analytics does not support inferential analysis. However, it helps in understanding data; data analytics also involves additional processes like machine learning (ML) and predictive data modeling to provide more accurate and beneficial for making data-driven decisions.
Data analysis is often employed in areas where understanding previous data and generating reports are essential, such as healthcare, finance, and marketing. It is beneficial for tracking real-time performance and reviewing previous outcomes. But, data analytics is more extensively employed in fields such as e-commerce, technology, and sports analytics. It is usually applied to predict past as well as latest trends, boost overall business performance, and optimize real-time plans. Due to its ability to enable long-term improvement and predictive and data-driven decision-making, data analytics is utilized more often.
Difference between Data Analysis and Data Analytics: Which Fits Better?
While both the data fields, data analysis and data analytics are essential for data-driven decision-making, they have different featrures. By discovering hidden data patterns and trends in past data, data analysis helps businesses in analysing and understanding previous performance. This is essential for developing reports and making real-time data-driven decisions.
However, another popular data field known as data analytics pushes one step further by predicting future data patterns and giving recommendations for instant actions. It helps businesses optimize and plan the most suitable strategy. While data analytics helps in promoting data-driven decisions and data analysis offers the basis of decisions, both are necessary. When using them combined, they aid businesses in making more data-driven business decisions and managing their competitiveness in a highly competitive industry that is rapidly transforming.
Wrapping Up,
In today’s rapidly transforming digital world infused by large volume of data, diffenciates data analysis from data analytics. While data analytics prioritises on collecting vital data from raw datasets addressing specific errors, data analysis uses significant data to make data-driven decision in real-time.
Hoping, I have elaborated on the key difference between data analysis and data analytics in this above article using very simple and easy terms. If you have any other differentiating factors in mind that I have missed mentioning in the above section, please feel free to add them in the comments section below.
So, commence your professional journey in IT by enrolling into these best courses after graduation from best institutions in India. The industry ready data analytics course of W3 Web School is highly profitable for students who wish to pursue their professional career in IT field.
Happy reading.