Benefits And Challenges Of GPU Cloud Servers For AI And Machine Learning
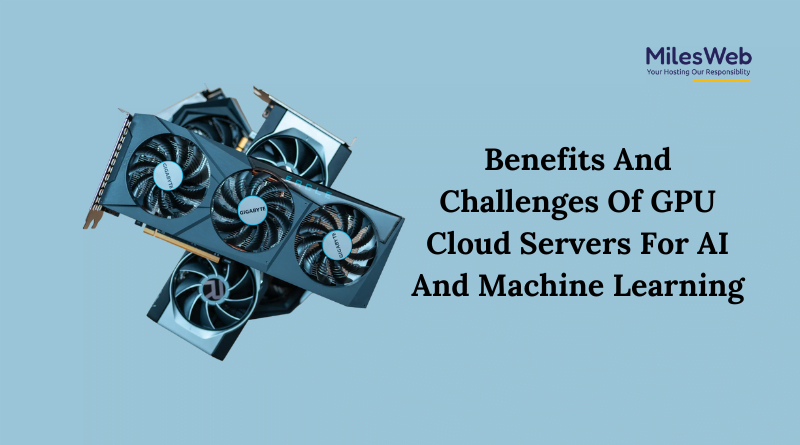
Engineering complex AI, i.e., artificial intelligence systems like LLMs (large language models) and deep learning networks, needs enormous computational power as their business operations expand.
GPU cloud server offer the required processing power to foster these developments in the AI sector. But having said that, which qualities of GPUs are best suited for this type of workload? And what is the GPU server price in India? The key to understanding this lies in the significant differences between GPUs and CPUs.
Both CPUs, i.e., central processing units, and GPUs, i.e., graphics processing units, are processors, and there’s a significant difference in their architectures. CPUs are leaders in sequential processing. Because of this, they’re perfectly suitable for operating systems and real-time process management.
However, their systematic approach encounters problems when working with huge datasets and complicated calculations needed for artificial intelligence model training. Because of this, GPUs are most commonly utilized in advanced technological transformations.
Originally designed for video game graphics, GPUs have now established themselves as basic elements in parallel data processing and play an integral part in today’s evolving online domain. Contrary to CPUs, GPUs can simultaneously manage various tasks, which makes them a perfect solution for speeding up the process of complicated AI algorithm development.
The GPU cloud servers have made it easy to develop complicated AI models. They’ve also minimized the learning curve and enabled the development of previously seen-as-impossible AI models. In this blog, we’ll thoroughly evaluate the fundamental aspects of GPU servers for AI and ML, together with their benefits and challenges, to provide you with the necessary knowledge.
Overview of GPU Cloud Servers
GPU servers are specialized computing devices built to speed up processing tasks that require parallel data computation. It can be utilized for deep learning, AI, and complex graphical computations.
Contrary to standard CPUs, GPUs can integrate one or more than one GPU to substantially improve the performance of certain computing procedures.
Advantages of GPU Cloud Servers for AI and ML
GPU servers provide unmatched performance for machine learning and AI by speeding up complicated parallel data processing tasks and facilitating swift AI training processes, resulting in more effective and flexible solutions.
Key benefits of GPU cloud servers for AI and machine learning include:
1. Enhanced Speed and Efficiency
GPUs can simultaneously process multiple tasks, which makes them one of the crucial elements of heavy-duty computing architectures. Contrary to CPUs, they’re perfect at parallel processing, which carries out tasks in sequential order. Due to these abilities, they can efficiently handle huge calculations that are needed for machine learning and AI.
Several GPUs can be utilized to offer substantially greater processing capabilities, which can minimize the time required for data processing and training models. This setup is best fit for huge or complex machine learning workloads because it divides complex tasks and processes in parallel.
As a result of these capabilities, their computational tasks can be accelerated and the performance of complicated AI models can be improved. They’ve thus drastically transformed AI and ML. Their parallel processing architecture establishes them as an exceptional choice for complex data processing needs in these sectors.
2. Increased Productivity and Scalability
These supercharged GPU servers significantly strengthen advanced computing and deep learning. They’ve got robust parallel computational capabilities and improved efficiency when handling massive data sets. These features are crucial for the most sought-after ML and AI applications.
Scalability enables GPUs to efficiently manage a higher number of requests, respond quickly, and avoid errors or slowdowns. They can reduce maintenance and operating costs while simultaneously facilitating more users, customers, and operations.
Increased productivity and efficiency are important for multiple reasons. First of all, it could reduce expenses, increase precision, avoid delays, and at the same time increase user satisfaction.
Efficiency can also enhance the scalability and reliability of an AI system by avoiding overloads, challenges, and limitations. Scalability is crucial for real-time AI technologies. In order to effectively deal with real-world issues of individuals and businesses, it is mandatory to respond by scaling the performance of AI technologies and infrastructure.
3. Affordable Solution
Even after their initial capital investment, due to their improved computational power, GPU cloud servers can minimize time and operating costs. Using a GPU server, labor-intensive processes can be completed in hours or days because of the quick training model and inference.
Additionally, multiple cloud providers sell VMs (virtual machines) with GPUs, allowing clients to easily access powerful computational resources without requiring large-scale initial capital investments. GPU power for AI and ML applications can be affordably used with the help of their pay-as-you-use model.
4. Expert Support for Complex AI Solutions
Robust computational powers are required for comprehensive neural networks and complex codes that are standard elements of the latest AI and ML technologies. These systems are perfectly suitable for backing up these modern-day tasks.
GPUs are utilized for example, in the process of deep learning model training, which consists of several parameters and layers. GPUs’ parallel processing abilities make it easy to train for these models and pave the way for developing complicated AI models.
They’re also necessary for critical evaluation activities, which include AI-powered decision-making processes or insights derived from the latest datasets. Some examples of real-time applications are voice assistants, smart recommendation solutions, and driverless cars that reap the advantage of accelerated computing processing.
These applications can work flawlessly and provide instant feedback.
5. Improved Research and Development Potential
GPU-dedicated servers are perfect due to their heavy computational operations, parallel processing abilities, superior performance, and reliability. They also provide customized hardware, powerful software support, and scalability. These types of servers will be important elements of AI and machine learning for the future.
These types of servers are also best for parallel processing in machine learning and AI applications since they customize data through parallel processing. Managing the sheer volume of data is necessary for deep learning model training.
Also, big data analytics is crucial since it enables businesses to make use of huge data sets from different sources to identify potential advantages and difficulties. They can also help businesses simplify processing tasks and increase productivity.
Challenges of GPU Cloud Servers
GPU cloud servers are highly significant for speeding up AI and ML projects; however, they’ve got their distinct problems. From tackling the problems related to increased costs and changing performance to assuring powerful security, these challenges should be handled effortlessly to get the most out of the GPU technology.
1. Financial Concerns
Purchasing GPUs, especially exclusive ones that are specifically built for heavy-duty computational workloads, can lead to huge expenses. Also, developing a multi-GPU system could lead to significant financial expenses. Which can be a monetary issue for small business owners or personal users.
2. Energy Consumption
Running GPU servers requires considerable electric power, leading to heftier processing costs and potential heat-related problems needing additional cooling to resolve overheating chances.
3. Software Integration
Specific software programs, applications, and algorithms aren’t naturally suited for GPU-powered computational processes. Even though standard platforms such as TensorFlow and PyTorch support GPUs, outdated codes or less prominent applications might not provide equivalent capabilities.
Adjusting to new codes and assuring accurate compatibility can require additional effort.
4. Storage Limitations
Contrary to traditional CPUs, GPUs mostly have minimum storage capacities. Huge dataset processing and complicated modeling techniques that require vast memory resources could face challenges in this case.
Effective memory management techniques and strategic efficiency improvement techniques help in resolving these issues.
5. Required Skill Set
Developing GPU-optimized applications requires knowledge of GPU programming, for example, CUDA or PenCL. Expertise in these complex architectures and associated syntaxes usually requires a significant amount of time to learn for those who know traditional CPU programming.
6. Transmission Latency
In a distributed system, the data transfer between GPUs and related hardware elements sometimes results in an RDS (rate-determining step). Also, increasing GPU performance might prove to be challenging until data transfer rates adjust to their advanced processing speed.
In this way, enhancing data transfer protocols and minimizing secondary communication is of utmost significance.
7. Expansion Constraints And Resource Optimization
Expanding GPU resources in distributed systems can lead to complicated scaling issues. Equalizing working load distributions among several GPUs or handling GPU allotments in shared environments requires efficient resource application to guarantee smooth operations.
Why Choose MilesWeb’s Top-Notch NVIDIA Cloud GPUs?
MilesWeb’s groundbreaking NVIDIA cloud GPU servers provide unmatched performance for heavy-duty applications and resource-intensive workloads. By implementing this leading-edge technology, our cloud GPUs offer excellent processing power, assuring quick processing and advanced graphics simulation.
This leads to a significant improvement in different applications like AI, ML, and data analysis. Using scalable solutions customized to your distinct needs, MilesWeb makes sure that you’re billed as per your actual resource usage, providing affordable solutions without affecting performance.
Their powerful server infrastructure ensures easy availability and dependability, making their NVIDIA cloud GPU the most preferred choice for enterprises looking to increase their processing capabilities and accomplish their long-term goals.
Conclusion
GPUs have comprehensively modernized computational efficiency, substantially improving the speed and performance of data processing for a multitude of applications. Their impact is significant and far-reaching without any deviation. Furthermore, their consistent development guarantees to drive significant advancements in the years ahead.